Analyzing Wheat Heads With Image Analysis and Machine Learning
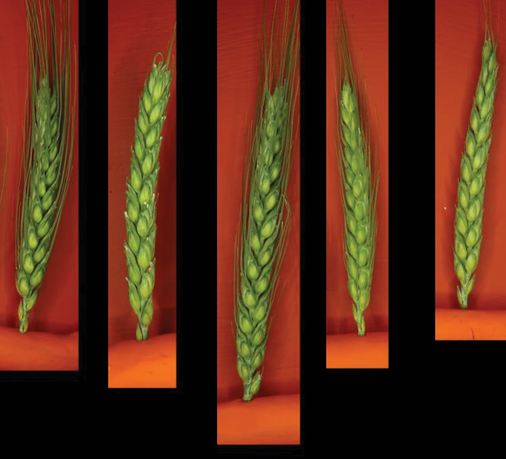
Plant breeders need large amounts of data to identify genetic components behind traits of interest. While advances in genotyping have made it easier to gather genomic data, measuring phenotypes can still be expensive, tedious, and laborious. Improving the speed and accuracy of phenotyping would increase the amount of data breeders could collect, which could improve research efforts. Image analysis and machine learning could improve the efficiency of measuring traits in plants.
Researchers collected wheat spikes from the field and imaged them in a controlled environment. They used these images to train and test a variety of machine‐learning algorithms, including previously developed models such as VGG16, ResNet152, and EfficientNetB7. These models were trained to estimate the number of spikelets on each wheat spike, a trait related to grain yield per spike. Some of the models were able to estimate spikelet number with high accuracy.
Discovery of accurate and reliable trait data analysis methods is crucial for creating efficient data collection systems in the plant sciences. While the process of developing the methods and training the models can be a large commitment, they are a necessary aspect of the phenotyping pipeline.
Adapted from
Hammers, M., Winn, Z. J., Ben‐Hur, A., Larkin, D., Murry, J., & Mason, R. E. (2023). Phenotyping and predicting wheat spike characteristics using image analysis and machine learning. The Plant Phenome Journal, 6, e20087. https://doi.org/10.1002/ppj2.20087
Text © . The authors. CC BY-NC-ND 4.0. Except where otherwise noted, images are subject to copyright. Any reuse without express permission from the copyright owner is prohibited.