Can Plant Breeding Ignore Genomic Selection?
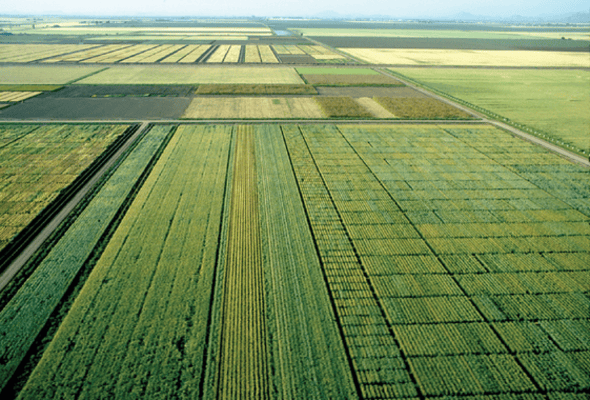
Genomic selection (GS) is a predictive methodology that revolutionized plant breeding by allowing breeders to select potential candidates before they are planted. In GS, a statistical machine‐learning model is trained with recorded phenotypic and genotypic information from a reference population and then deployed to make predictions regarding individuals for which only genotypic information is available. It is implemented in many crops, but application of GS is complex because successful implementation is determined by the predictive accuracy of the machine‐learning methods employed.
To explore if genomic marker information improves prediction accuracy in plant‐breeding trials, researchers compared the performance of prediction models using and not using genomic information in 14 datasets. In some datasets, the gain in prediction accuracy when including genomic information was almost null, but in others datasets, the gain was more than 50%. Across traits, environments, and datasets, the researchers found that the gain in prediction accuracy using genomics was around 46.1%.
These findings corroborate that the quality of the genomic information and a moderate to high degree of relatedness among the individuals in the training and testing set is of paramount importance to take full advantage of GS.
Adapted from
Montesinos‐López, O. A., Bentley, A. R., Saint Pierre, C., Crespo‐Herrera, L., Rebollar‐Ruellas, L., Valladares‐Celis, P. E., Lillemo, M., Montesinos‐López, A., & Crossa, J. (2023). Efficacy of plant breeding using genomic information. The Plant Genome, 16, e20346. https://doi.org/10.1002/tpg2.20346
Text © . The authors. CC BY-NC-ND 4.0. Except where otherwise noted, images are subject to copyright. Any reuse without express permission from the copyright owner is prohibited.