Deep learning delivers accurate dollar spot detection across turfgrass species
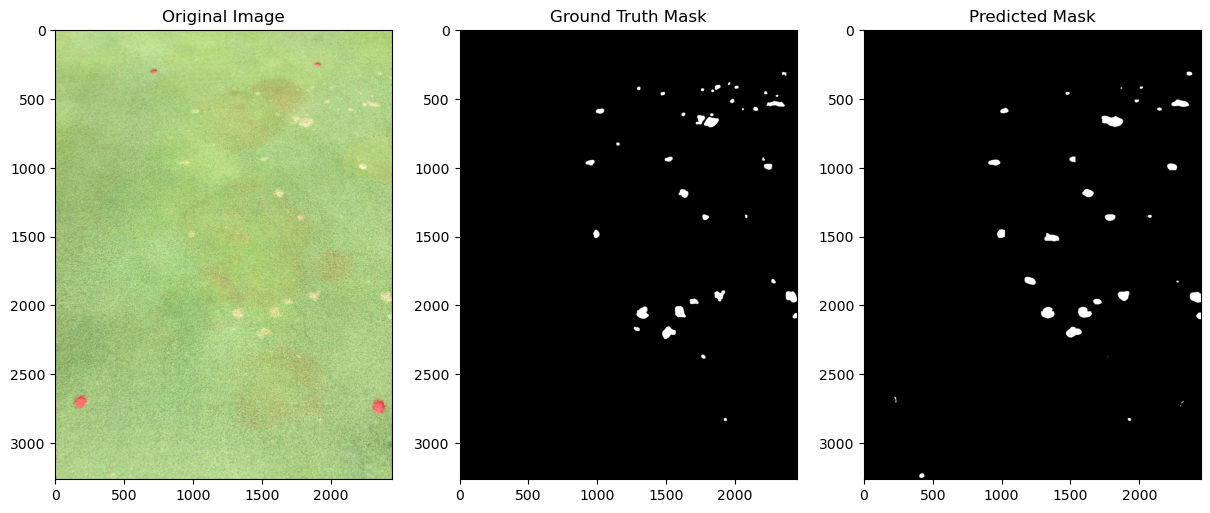
Turfgrass is critical to the aesthetic and functional quality of landscapes and sports fields, but diseases such as dollar spot (Clarireedia spp.) present ongoing challenges. This fungal disease affects a range of turfgrass species, causing economic losses through turf damage and increased management expenditures. Traditional methods for assessing dollar spot are time and labor intensive, often relying on visual estimations to quantify disease spread, which introduces subjectivity and inconsistency.
In a recent study in Crop Science, researchers aimed to address these limitations by utilizing the DeepLabV3+ deep learning model to detect and quantify dollar spot from field images. They fine-tuned the model to segment images at the pixel level, accurately identifying dollar spot across multiple turfgrass species, mowing heights, and stages of disease development. The model achieved a mean pixel accuracy of 97% with an average inference time of 6 milliseconds per image, offering faster and more consistent assessments compared with traditional methods.
These results highlight the potential for deep learning models to transform disease management in turfgrass by providing faster, more accurate, and consistent methods for disease quantification, offering broad implications for improving precision in turfgrass care.
Dig deeper
Kitchin, E. C. A., Sneed, H. J., & McCall, D. S. (2024). Leveraging deep learning for dollar spot detection and quantification in turfgrass. Crop Science. https://doi.org/10.1002/csc2.21329
Text © . The authors. CC BY-NC-ND 4.0. Except where otherwise noted, images are subject to copyright. Any reuse without express permission from the copyright owner is prohibited.