Using machine learning to estimate soil hydraulic parameters of layered profiles
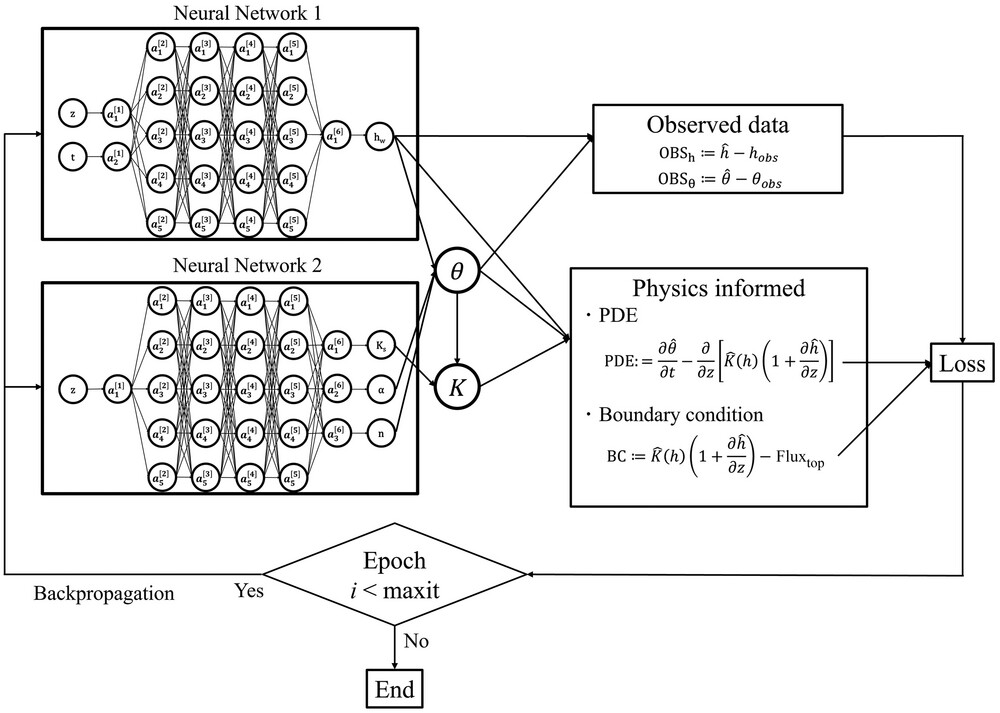
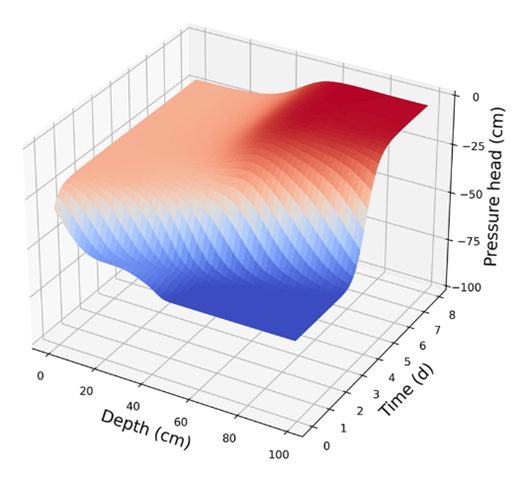
Deep learning has become inseparable from our daily lives and is expected to be used more widely in agricultural sciences and practices in the future. While physics-informed neural networks (PINNs), a type of deep learning that allows enforcing physical constraints, have been used to successfully analyze soil water dynamics, further research results are required for application to real field problems.
Using PINNs to estimate spatially varying soil hydraulic properties from observed soil water pressure head and water content data is attractive because of their flexibility in computation settings compared with standard numerical approaches. By combining two PINNs, one for predicting soil water dynamics and the other for predicting soil hydraulic properties, researchers at Tokyo University of Agriculture and Technology were able to predict the distributions of soil hydraulic properties in addition to changes in soil water content within the layered soil profiles.
Although the proposed PINNs were tested only for one-dimensional cases, they can be further extended to estimate spatial distributions of soil hydraulic properties in two- and three-dimensional domains. This can significantly broaden the ability to predict mass and energy transport in heterogenous field soils and to optimize water and nutrient management for sustainable agriculture.
Dig deeper
Oikawa, K., & Saito, H. (2024). Inverse analysis of soil hydraulic parameters of layered soil profiles using physics-informed neural networks with unsaturated water flow models. Vadose Zone Journal, 23, e20375. https://doi.org/10.1002/vzj2.20375
Text © . The authors. CC BY-NC-ND 4.0. Except where otherwise noted, images are subject to copyright. Any reuse without express permission from the copyright owner is prohibited.